Predicting the trends of RAF based on EHR and claims data. See the case study
case study highlight
Achieved 95% Treatment in Place with Custom Virtual Care Ecosystem
A large value-based care provider, faced CMS reimbursement challenges due to coding gaps. Complex growth and tech hindered patient care. Addressing gaps was vital for improved patient care and reimbursement.
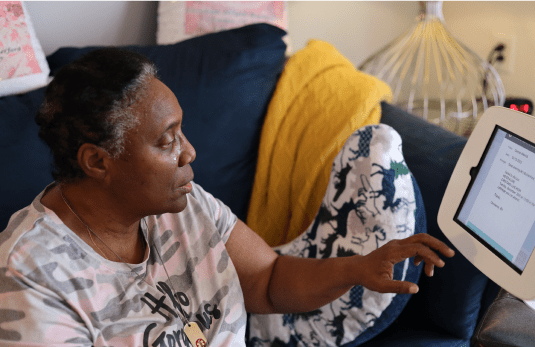