RAF Score (Coding AI)
New Risk Adjustment Factor models for reliable scoring
healthcare, user research, user experience, product design + development
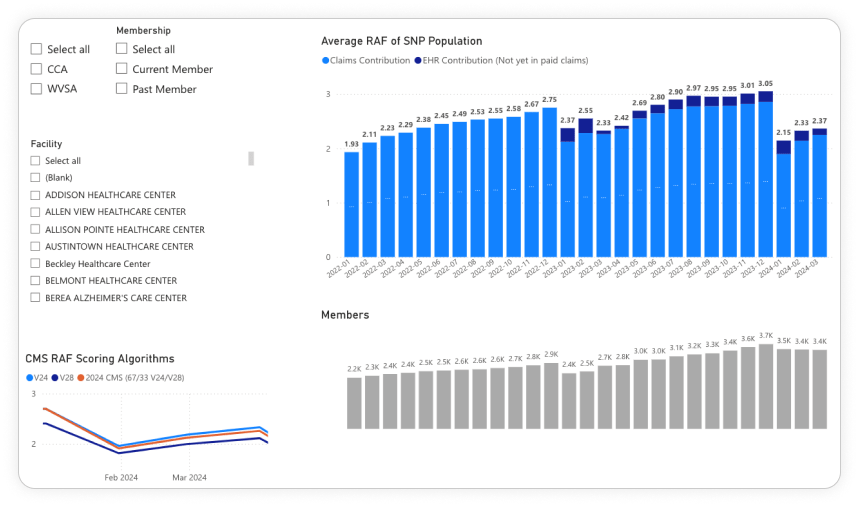
overview
Custom Risk Adjustment Factor solution delivers reliable scoring model
Best in class organizations delivering value based care are required to manage patient risk levels. Inability to do so leads to inaccurate risk scores, declining reimbursements, and sub optimal patient care. A targeted engagement to shore up these key elements led this organization back to delivering value based care at the highest levels.
A large value-based care provider, serving 20K+ patients in 7 states, faced CMS reimbursement challenges due to coding gaps. Complex growth and tech hindered patient care, requiring better coordination among 200+ providers. Addressing gaps was vital for improved patient care and reimbursement.
industries
- Digital health
- Social Determinants of Health
services
- Product strategy
- UX research & design
- Custom software development
- Strategic business advisory
- Competitive market analysis
- Capability differentiation
- Distribution strategy
- Product & market thought leadership
- Product roadmapping
Accurate RAF Score
Risk Assessment that is reflective of a comprehensive view of all chronic & acute patient conditions.
Automated Workflow
Personalized recommendations delivered by an automated AI/ML model assessing multiple datasets.
Optimized Reimbursement
Accurate RAF score leads to accurate reimbursement needed to deliver optimal care for the patient.
The challenge
Ensure accurate RAF scores reflecting all conditions, prompt capture of chronic/episodic conditions, timely clinician assessments, and compliant, comprehensive data for precise reimbursement.
Impact
Higher recapture rates at ICD/HCC levels improved risk score accuracy, reducing hospitalizations. Clinicians gained holistic patient views, leading to integrated care planning. Accurate coding minimized errors, optimizing CMS reimbursement for ACO
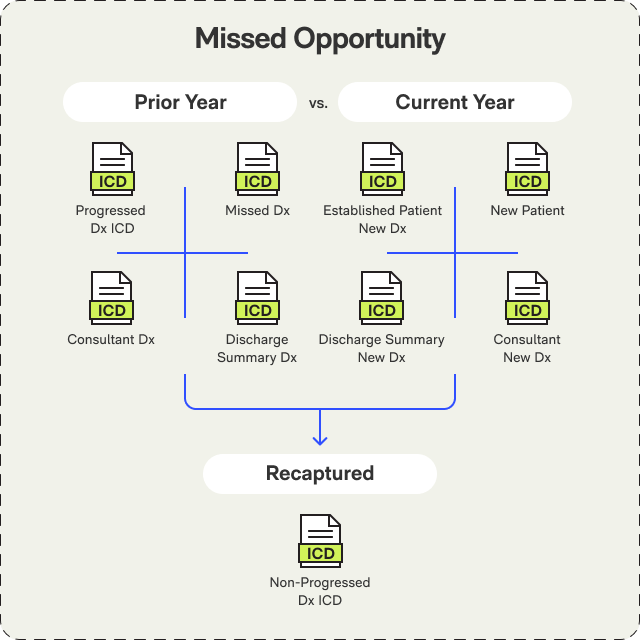
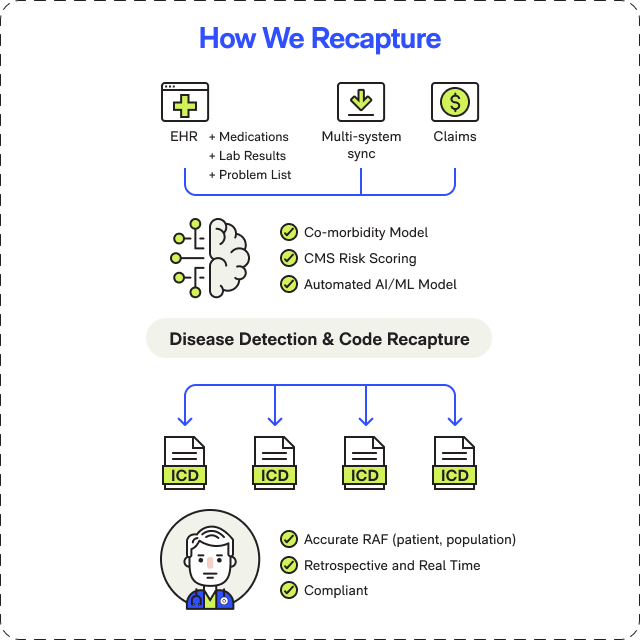
What we did
Evaluated Inputs
Team carefully assessed and determined the list of inputs that had to be evaluated:
- Prior patient diagnosis history through Gehrimed and PCC
- Prior patient assessments like HRA’s (Health Risk Assessments), MDS (Minimum Data Set), etc.
- Medication records
- Claims data going back multiple years
- Lab records
Analyzed Inputs
Multiple models leveraging AI were developed to analyse the inputs:
- Co-morbidity models across population and population subsets
- Generative AI model to recommend potential conditions for clinical feedback
- Peer reviewed CMS scoring model
Provided Targeted Recommendations
- Custom UI was created to deliver recommendation within clinician workflow
- Clinician feedback on dropped and probable diagnosis were ingested real time
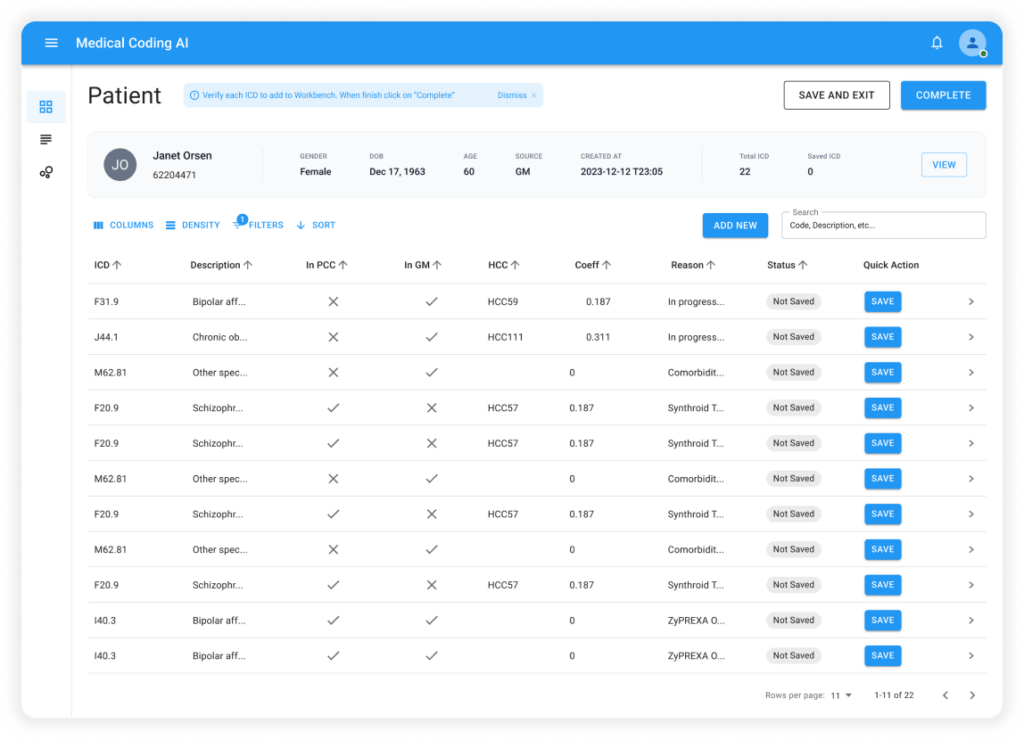
Industry Frameworks, Systems, Data Standards
- CMS-HSS Risk Adjust Models
- ACO, SNP Population Health
- RxNorm / RxClass
- Claims Analytics
- ICD-10 Coding
Deliverables
- Detailed documentation of current and future state covering data, architecture, insights, reports, UI
- All code for ML and AI models
- Co-morbidity model
- CMS risk scoring model updated with the latest CMS codes
- Custom UI to feed AI recommendations and ingest clinician feedback
- Integration into Gehrimed Workflow